training common sense
[[training-common-sense-day-2]] (friday)
[[training-common-sense-day-3]] (saturday)
[[training-common-sense-day-4]] (monday)
[[training-common-sense-day-5]] (tuesday)
---------------------------------------------------------
links
earlier notes:
the Annotater @ Cqrrelations, Jan. 2015
---------------------------------------------------------
git & the critical-fork-folder
to clone the Pattern 2.6 critical-fork folder
- git clone USERNAME@10.9.8.7:/home/bare_git_repositories/pattern-2.6-critical-fork/
---------------------------------------------------------
* shift -->
- before, traditionally : thinking in categories, researching for the truth through questionaires with predefined categories
- now, big data : thinking in raw data, natural 'truths', the truth is out there (in the raw data) just undiscovered
- * but... categorisation is still present in data-mining, but more hidden, and therefor harder grasp
- * still there are categories, but they are often disguished as math
* co-intelligence between humans and machines
* there is a lot of un-rawness to this data
* repeat the model untill the model is true
- example: history of western classical music doesn't involve woman, a comment was: but i looked, there are no
- example: alternative scientific truth searching methods --> work on (non) common sense valid truths
* [how]? [you]? [write]?
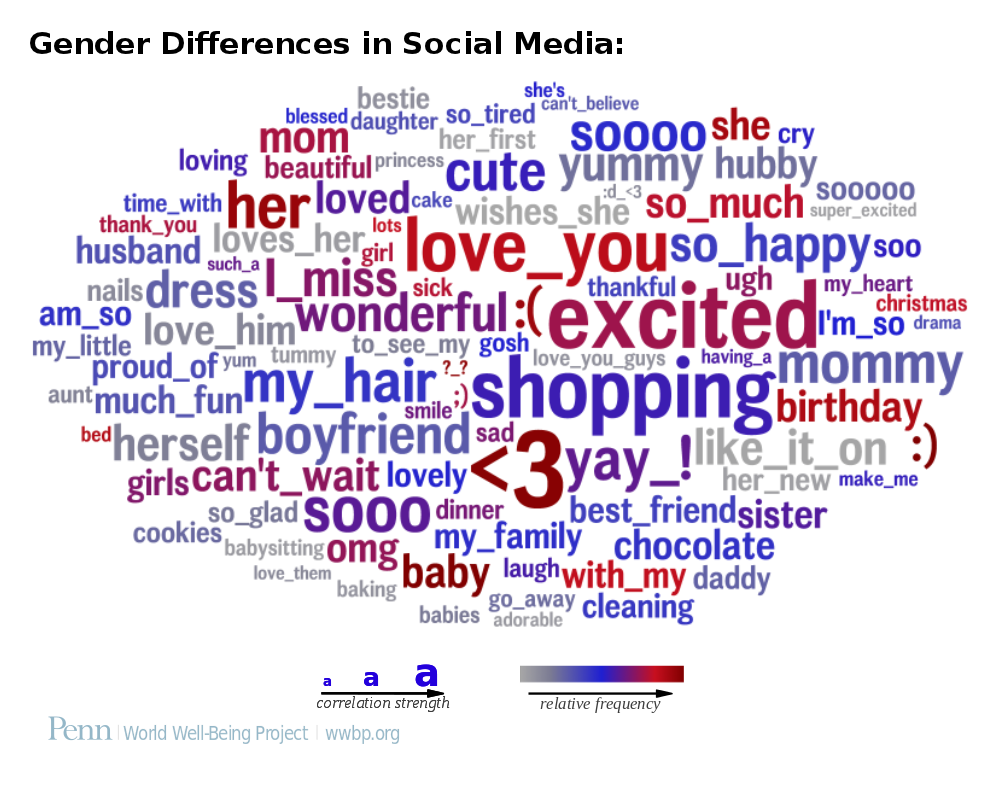
../images/gender-female-most-common-words_natural-result.png
from: the article Toward personality insights from language exploration in social media
door ut te betalen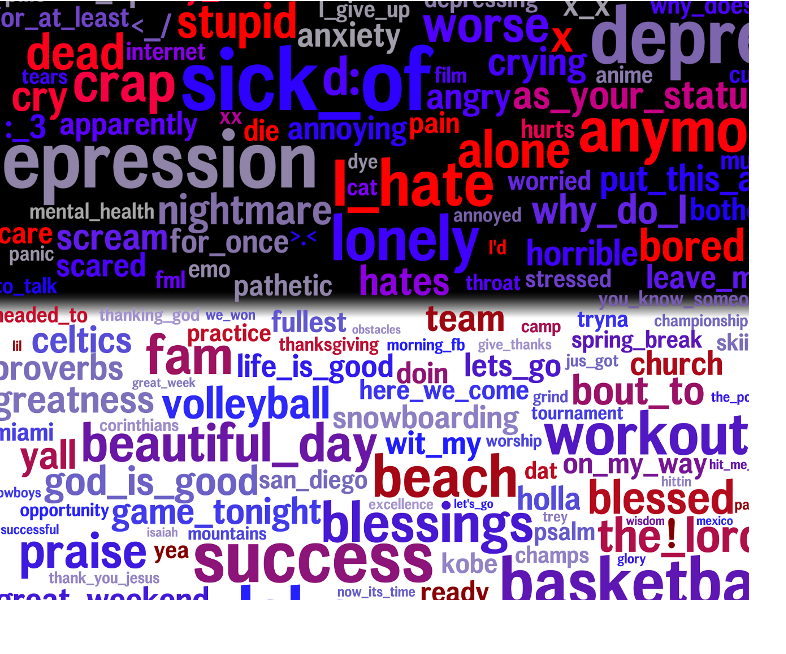
../images/article7.png
machine categorisation of positive / negative words
http://www.wwbp.org/data.html#refinement , from the article: Personality, gender, and age in the language of social media: The Open-Vocabulary Approach
looking at applications
Facebook messages Gender/Age profiles
Hedonometer --> http://hedonometer.org/
going to Knowledge Discovery in Data steps, by looking at Pattern
step 1 --> data collection
step 2 --> data preparation
step 3 --> data mining
step 4 --> interpretation
step 5 --> determine actions
Pattern
created at CLiPS, a research project at the University of Antwerp
http://www.clips.ua.ac.be/pages/pattern
annotating the presentation/
dealing with large amounts of data and triyng making senso of that
big data: the idea of looking at knowledge through categories
but its also raw data, which machine can 'read' through without thinking in relation to these categories (looking for truth)
the crudeness of the presented results: what does that mean? (gender differences in social medias)
what does it mean?
we wanto to look at:
- data collection (thier technological as well as social context)
- data preparation (look at the rough raw data and its next 'use')
- data mining (looking into mathematical models, graphs; the translation from linguistic models to mathematical clusters; looking at different projections, what are the differencies? the 'suitability' of the models in relation to the outcomes)
-
-
what we want to do:
- look in actual text mining programs, look and discuss what happens
- look at documentation on the collection/ing of this datas (exuses, apologies about the political/social problematics expressed in the 'truthfullness' of the mathematical results) - revealing subtleties
- the research on the data mining is very close to the text generation project
example:smilies-->not recognized as meaningful
meaningfulness of cleaning data (we know it is-like cleaning toilets- but we don't do it)
use facebook data to train next mining process - 'self-fulfilling prophecy' of common sense
data-mining->"the" step, but actually just a small part of the process
mathematical model--->truth finding moment-->the machine performance
with enough time, with enough data...
compare first results with standard, most of the time human-produced data
e.g. libraries of positivity/negativity online
The best material model of a cat is another, or preferably the same, cat.
Philosophy of Science 1945. (Wiener)
Project IMB: you are what your write on Twitter: http://venturebeat.com/2013/10/08/ibm-researcher-can-decipher-your-personality-in-200-tweets/
Suggested references
An article that poses questions and reflections on methodology applied in research. They obtain "valid cientific" results that have no common sense at all. The abstract is quite inspiring
http://www0.cs.ucl.ac.uk/staff/m.slater/Papers/colourful.pdf
ConceptNet aims to give computers access to common-sense knowledge, the kind of information that ordinary people know but usually leave unstated: http://conceptnet5.media.mit.edu/
Unfortunately, common sense is not yet within reach for this 4-year old: http://www.extremetech.com/extreme/161383-artificial-intelligence-has-the-verbal-skills-of-a-four-year-old-still-no-common-sense